Turning "Poop" Into Podcasts: An AI-Powered Approach To Data Analysis
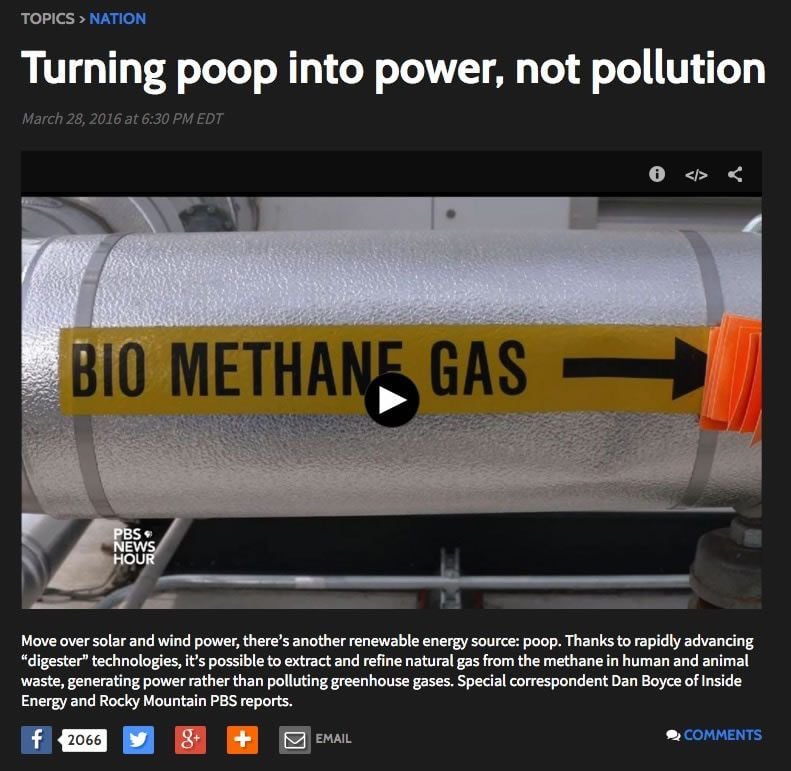
Table of Contents
The Challenge of Waste Data Analysis
Waste data analysis presents significant hurdles. The information is often unstructured, incomplete, and inconsistent, making traditional analysis methods incredibly challenging. This complexity stems from several key factors:
- Lack of standardized data collection methods across different regions: Different cities and countries employ varying methods for collecting and recording waste data, leading to inconsistencies and making comparisons difficult.
- Inconsistent waste composition reporting: The way waste composition is reported varies widely, hindering accurate analysis of material flows and recycling rates. Precise categorization is crucial for effective waste management strategies.
- Difficulties in real-time data monitoring: Lack of real-time data monitoring makes it difficult to respond quickly to changes in waste generation or identify potential problems.
- Limited accessibility to comprehensive datasets: Access to complete and reliable waste datasets is often restricted, limiting the scope of analysis and hindering the development of effective solutions.
These challenges significantly hinder effective waste management strategies. Without a clear understanding of waste generation patterns, composition, and flows, it's impossible to optimize recycling programs, improve landfill management, or develop effective waste reduction initiatives. This is where AI-powered data analysis of waste data steps in to provide solutions.
How AI Solves the Problem
AI offers powerful tools to overcome the limitations of traditional waste data analysis. Machine learning algorithms excel at processing large, complex datasets, identifying hidden patterns and trends that would be impossible to detect manually. This capability is crucial for unlocking the potential of waste data.
Specifically, several AI techniques are proving invaluable:
- Machine learning algorithms: These algorithms can identify correlations and predict future waste generation based on historical data and external factors (e.g., population growth, seasonal variations).
- Natural Language Processing (NLP): NLP allows the analysis of textual data from waste reports and surveys, extracting valuable insights that might be missed by manual review. This is especially useful for understanding public perception of waste management initiatives.
These methods allow for significant improvements in various aspects of waste management:
- Predictive modeling for waste generation forecasting: Accurate forecasting allows for proactive resource allocation and optimized waste collection routes.
- Improved waste sorting and recycling optimization: AI-driven systems can analyze the composition of waste streams in real-time, optimizing sorting processes and maximizing recycling rates.
- Real-time monitoring of landfill capacity: AI can monitor landfill levels, predict when they'll reach capacity, and optimize landfill space utilization.
- Identification of emerging waste streams and environmental threats: By analyzing trends in waste composition, AI can help identify new waste streams and potential environmental hazards.
Specific AI Techniques
Let's delve deeper into specific AI techniques used in waste data analysis:
- Deep learning for image recognition of waste materials: Deep learning algorithms can analyze images of waste to identify different materials with high accuracy, significantly improving the efficiency of automated sorting systems.
- Time series analysis for identifying seasonal variations in waste generation: Analyzing time series data reveals seasonal patterns, allowing for more effective resource allocation and predictive maintenance of equipment.
- Clustering algorithms to group similar waste types for efficient management: Clustering helps to identify similar waste streams, facilitating efficient collection, processing, and disposal strategies.
Transforming Data into Actionable Insights (The "Podcast" Analogy)
The power of AI-powered data analysis of waste data lies not only in its analytical capabilities but also in its ability to communicate complex insights effectively. The "podcast" analogy highlights the importance of translating raw data into easily digestible information. This is crucial for informing decision-making at all levels.
AI-driven insights can be effectively communicated through various channels:
- Interactive dashboards visualizing key waste metrics: Dashboards provide a clear overview of key performance indicators (KPIs), enabling stakeholders to monitor progress and identify areas for improvement.
- Automated reports summarizing key findings: Regularly generated reports provide concise summaries of key findings, facilitating data-driven decision-making.
- Data-driven presentations for stakeholders: Presentations using compelling visuals and clear narratives effectively communicate insights to stakeholders, including policymakers, waste management companies, and the public.
- Public awareness campaigns using easily understood information: Making complex data accessible to the public through infographics, social media campaigns, and public forums can improve community engagement and participation in waste reduction programs.
Conclusion
AI-powered data analysis of waste data is revolutionizing waste management by turning mountains of seemingly unusable data into actionable insights. By employing machine learning, NLP, and other advanced techniques, we can overcome the inherent challenges of waste data analysis and create more efficient, sustainable waste management systems. The "podcast" analogy highlights the importance of transforming complex data into readily consumable information for a variety of audiences.
Ready to turn your own "poop" into podcasts? Explore the possibilities of AI-powered data analysis in waste management and discover how you can contribute to a cleaner, more sustainable future. Learn more about AI solutions for waste data analysis today!
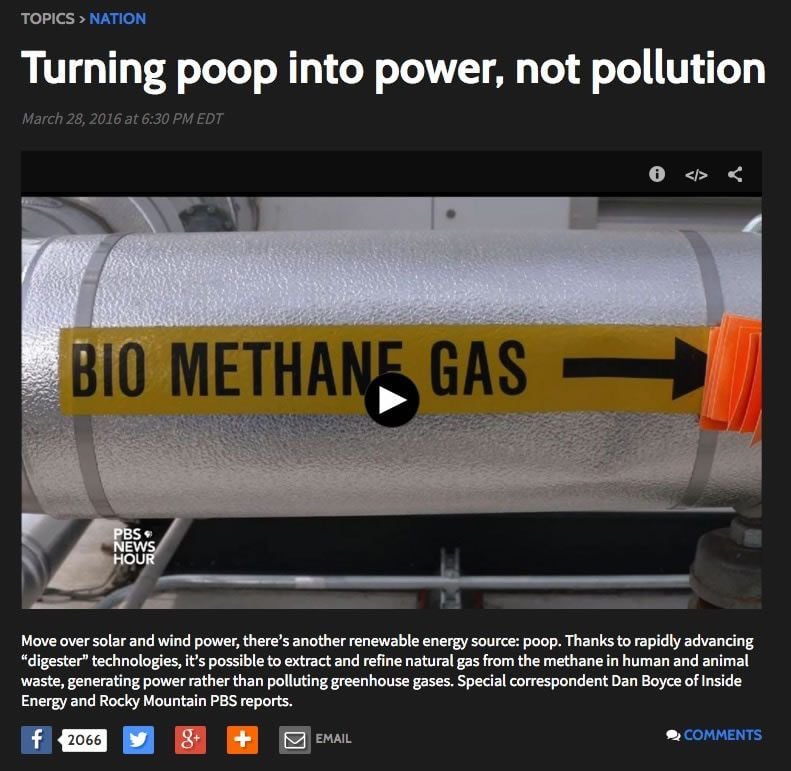
Featured Posts
-
Broadcoms Proposed V Mware Price Hike A 1 050 Increase For At And T
Apr 24, 2025 -
Is A Chinese Buyout Firm Selling Chip Tester Utac
Apr 24, 2025 -
Trump Administration Policies And Their Impact On Elite University Funding
Apr 24, 2025 -
John Travolta Honors Late Son Jetts 33rd Birthday With Moving Photo
Apr 24, 2025 -
La Palisades Wildfires Which Celebrities Lost Their Homes
Apr 24, 2025