AI And The Podcast Revolution: Analyzing Repetitive Scatological Documents
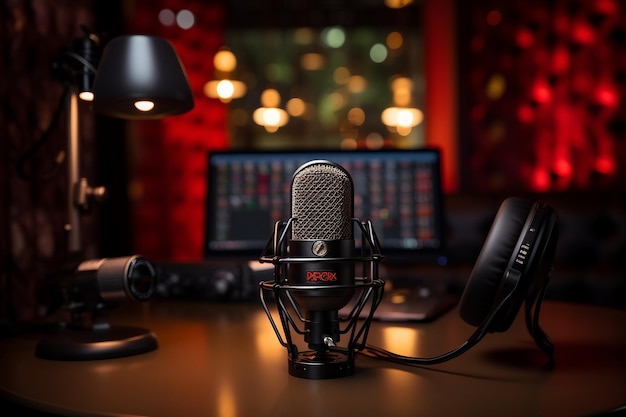
Table of Contents
AI-Powered Transcription and Analysis of Repetitive Scatological Documents
Analyzing RSDs traditionally involves laborious manual transcription and analysis, a time-consuming and potentially error-prone process. AI is rapidly changing this.
Speed and Efficiency
AI-powered transcription tools offer a significant advantage in speed and efficiency. They can process large audio files far quicker than human transcribers, freeing up valuable time and resources.
- Faster processing of large audio files: AI can transcribe hours of audio in minutes, drastically reducing processing time.
- Reduced human error in transcription: Automated transcription minimizes the risk of human error, leading to more accurate and reliable data for analysis.
- Cost-effective solution: AI transcription is often a more cost-effective solution than hiring multiple human transcribers, particularly for large-scale projects. This makes AI Podcast Analysis more accessible to researchers with limited budgets.
Identifying Patterns and Trends in RSDs
Beyond simple transcription, AI algorithms excel at identifying subtle patterns and trends within RSDs that might be missed by human analysts. This capability unlocks valuable insights.
- Sentiment analysis: AI can determine the overall emotional tone of the documents, revealing shifts in sentiment over time or across different speakers.
- Topic modeling: Sophisticated algorithms can identify recurring themes and subjects within the RSDs, providing a structured overview of the content.
- Anomaly detection: AI can highlight unusual or significant occurrences within the data, potentially pointing towards crucial events or shifts in patterns. This is particularly useful in identifying outliers in the AI Podcast Analysis process.
AI-Driven Content Categorization and Organization
The sheer volume of data generated by podcasts, especially when dealing with RSDs, necessitates efficient organization and categorization. AI provides the solution.
Automated Tagging and Metadata
AI can automatically tag and categorize RSDs based on their content, significantly improving searchability and organization.
- Improved searchability and organization: Automated tagging makes it easier to find specific data points within a large dataset, streamlining the research process.
- Facilitates collaborative research: Standardized tagging allows for easier collaboration and data sharing among researchers working on similar projects.
- Reduces manual categorization: AI automates a tedious task, freeing up researchers to focus on higher-level analysis.
Building a Comprehensive Database of RSDs
AI can be instrumental in building and maintaining comprehensive, searchable databases of RSDs from various sources.
- Centralized repository: AI facilitates the creation of a centralized repository, making it easy to access and compare data across different sources.
- Advanced data analysis: A well-organized database enables more sophisticated data analysis, including cross-referencing and trend identification.
- Supports long-term research: This database supports long-term research initiatives and ensures the preservation of valuable data.
Ethical Considerations in AI-Driven RSD Analysis
While AI offers powerful tools, ethical considerations are paramount, particularly when dealing with sensitive data like RSDs.
Data Privacy and Security
Protecting the privacy of individuals mentioned in RSDs is crucial.
- Anonymization techniques: Implementing anonymization techniques is essential to protect sensitive information.
- Secure storage and access control: Secure storage and robust access control mechanisms are necessary to prevent unauthorized access to the data.
- Compliance with regulations: Adhering to data privacy regulations like GDPR and CCPA is vital.
Bias Detection and Mitigation
AI algorithms can reflect existing biases in the data they are trained on.
- Regular auditing: Regular auditing of AI algorithms for bias is essential to ensure fairness and objectivity.
- Diverse datasets: Training AI models on diverse and representative datasets helps to mitigate bias.
- Bias mitigation methods: Implementing methods to actively mitigate bias in the analysis process is crucial for ensuring reliable results.
Conclusion
The integration of AI into podcast analysis, particularly concerning repetitive scatological documents, presents remarkable opportunities. From streamlining transcription and analysis to enabling advanced pattern recognition and ethical data handling, AI tools are transforming the research landscape. By leveraging the power of AI, researchers can gain unprecedented insights into these often-overlooked data sources, furthering our understanding of complex social and cultural phenomena. Embrace the AI and Podcast Revolution and start exploring the potential of AI-driven analysis for your RSD research today! Explore the latest AI Podcast Analysis tools and techniques to revolutionize your research workflow.
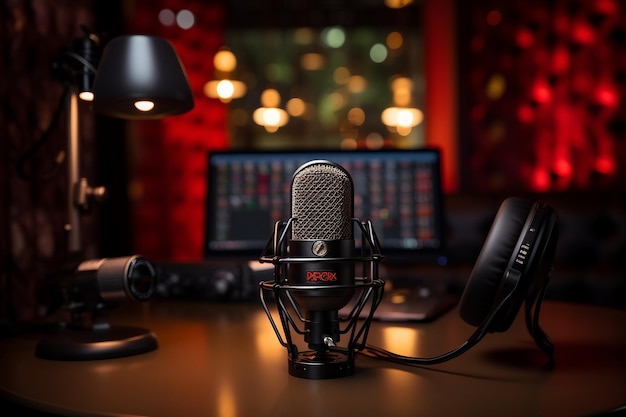
Featured Posts
-
Elite Universities Adapting To Shifting Political Landscapes And Funding Models
Apr 24, 2025 -
Canadian Dollars Complex Forex Performance A Current Overview
Apr 24, 2025 -
Thursday February 20th Bold And The Beautiful Spoilers Liam Steffy And Finns Upcoming Drama
Apr 24, 2025 -
India Market Analysis Tailwinds And The Niftys Bullish Momentum
Apr 24, 2025 -
Stock Market Gains Tariff Hopes Fuel Dow Nasdaq And S And P 500 Surge
Apr 24, 2025